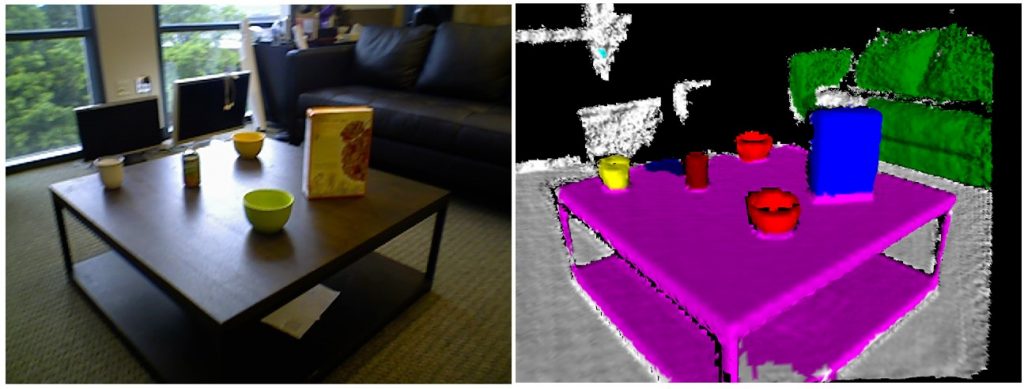
Understand the 3D world from 2D images
Course Information
Term: Spring 2023
Class Level: Graduate
Activity Type: Lecture
Days & Times: Monday & Wednesday 1:00 PM – 2:15 PM
Location: ECSW 3.210
Instructor: Prof. Yu Xiang
Office Location: ECSS 4.702
Office Hours: Monday & Wednesday 3:30PM – 4:30 PM
Teaching Assistant: Jishnu P
Office Hours: Tuesday 3:00PM – 4:00 PM
All the course materials can be found here.
Course Description
Theory and practice of computer vision. Provides in-depth overview of computer vision, including geometric primitives and transformations, camera models, image features, epipolar geometry and stereo, structure from motion and SLAM, 3D reconstruction, variations of modern neural networks and various recognition problems such as object detection, semantic segmentation, and human pose estimation.
Textbooks
Richard Szeliski. Computer Vision: Algorithms and Applications. 2011th Edition. Springer.
ISBN-13: 978-1848829343
ISBN-10: 1848829345
Second Edition Draft
David Forsyth, Jean Ponce. Computer Vision: A Modern Approach, 2nd Edition. Pearson, 2011. (Optional)
ISBN: 9789332550117
Richard Hartley. Multiple View Geometry in Computer Vision, 2nd Edition. Cambridge University Press, 2004. (Optional)
ISBN-13: 978-0521540513
ISBN-10: 0521540518
Grading Policy
- Homework (50%)
- Assignment 1 (10%)
- Assignment 2 (10%)
- Assignment 3 (10%)
- Assignment 4 (10%)
- Assignment 5 (10%)
- Team Project (45%)
- Project proposal (5%)
- Project mid-term report (10%)
- Project presentation (15%)
- Project final report (15%)
- In-class Activity (5%)
Project
- Project proposal description (PDF)
- Project mid-term report requirement (PDF)
- Project presentation and final report requirement (PDF)
Homework
- Assignment 1 (PDF, programming)
- Assignment 2 (PDF, programming)
- Assignment 3 (PDF, programming)
- Assignment 4 (PDF, programming)
- Assignment 5 (PDF, programming)
Learning from the “Cracker Box”
Guest Lecturer
Dr. Saining Xie from NYU will talk about representation learning and visual recognition on 4/26/2023.
Title: Scalable Visual Pre-training: Past, Present, and Paths Forward
Abstract
The remarkable visual recognition abilities of humans are rooted in a strong biological and cognitive foundation. In the past decade, deep learning has made significant strides across numerous domains, with representation learning playing a key role in this progress. This area focuses on learning efficient, accurate, and robust representations from raw data that can be utilized by a downstream classifier or predictor. Contemporary deep learning systems consist of two intertwined core components: 1) neural network architectures and 2) representation learning algorithms. In this lecture, we will explore several studies in both areas. We will delve into modern network design principles and their impact on the scaling behavior of ConvNets and Vision Transformers. We will also examine our endeavors to transcend the conventional supervised learning paradigm, showcasing how self-supervised visual representation learning can surpass supervised learning in various visual recognition tasks. Our discussion will cover a range of vision application domains and modalities (e.g., 2D images, 3D scenes and vision+language), illustrating the connections between techniques tailored for different input modalities and shedding light on the distinct challenges each modality presents. Lastly, we will address several critical challenges and opportunities that the “Large Pre-trained Model” era presents for computer vision research.
Lectures
Date | Topic |
Week 1, 1/16 | Martin Luther King Day |
Week 1, 1/18, Lecture 1 | Introduction to Computer Vision (slides) |
Week 2, 1/23, Lecture 2 | Image Formulation: Geometric Primitives and Transformations (slides) |
Week 2, 1/25, Lecture 3 | Image Formulation: 3D Rotations (slides) |
Week 3, 1/30, Lecture 4 | Image Formulation: Camera Models (slides) |
Week 3, 2/1 | Cancelled due to weather conditions![]() |
Week 4, 2/6, Lecture 5 | Image Formulation: Visual Rendering: Vertex Transforms (slides) |
Week 4, 2/8, Lecture 6 | Image Formulation: Visual Rendering: Rasterization, Lighting and Shading, Fragment Processing (slides) |
Week 5, 2/13, Lecture 7 | Feature Detection and Matching: Keypoint Features: Image Convolution and Harris Corner Detector (slides) |
Week 5, 2/15, Lecture 8 | Feature Detection and Matching: Keypoint Features: Scale Invariance and SIFT (slides) |
Week 6, 2/20, Lecture 9 | Feature Detection and Matching: Edges, Contours and Lines (slides) |
Week 6, 2/22, Lecture 10 | 3D Vision: Camera Calibration and Pose Estimation (slides) |
Week 7, 2/27, Lecture 11 | 3D Vision: Epipolar Geometry and Stereo (slides) |
Week 7, 3/1, Lecture 12 | 3D Vision: Structure from Motion and SLAM (slides) |
Week 8, 3/6, Lecture 13 | 3D Vision: 3D Reconstruction (slides) |
Week 8, 3/8, Lecture 14 | Deep Learning: Convolutional Neural Networks I (slides) |
Week 9, 3/13 | Spring Break |
Week 9, 3/15 | Spring Break |
Week 10, 3/20, Lecture 15 | Deep Learning: Convolutional Neural Networks II (slides) |
Week 10, 3/22, Lecture 16 | Deep Learning: Recurrent Neural Networks (slides) |
Week 11, 3/27, Lecture 17 | Deep Learning: Transformers (slides) |
Week 11, 3/29, Lecture 18 | Deep Learning: Generative Neural Networks (slides) |
Week 12, 4/3, Lecture 19 | Deep Learning: Neural Networks for 3D Data (slides) |
Week 12, 4/5, Lecture 20 | Recognition: Visual Representation Learning (slides) |
Week 13, 4/10, Lecture 21 | Recognition: Optical Flow and Correspondences (slides) |
Week 13, 4/12, Lecture 22 | Recognition: Object Detection (slides) |
Week 14, 4/17, Lecture 23 | Recognition: Semantic Segmentation (slides) |
Week, 14, 4/19, Lecture 24 | Recognition: Pose Estimation of Objects, Humans and Hands (slides) |
Week 15, 4/24, Lecture 25 | Recognition: Images and Languages (slides) |
Week 15, 4/26 | Guest Lecture: Dr. Saining Xie Scalable Visual Pre-training: Past, Present, and Paths Forward (slides) |
Week 16, 5/1 | Project Presentation I Group 2: Lane and Obstacle Detection for Autonomous Vehicles (slides, demo) Group 3: Human Pose Estimation based Posture Corrector (slides, demo) Group 6: Image Search Engine (slides) Group 7: Hand Gesture Recognition for Interaction with Computers (slides) Group 8: Verification of Identity using Triplet Network (slides) Group 9: Memento: Object Detection and Tracking for Memory Recall (slides, demo) Group 10: Vehicle Detection, Classification and Counting (slides) Group 11: Human Movement Analysis for Sports Performance Evaluation (slides, demo) |
Week 16, 5/3 | Project Presentation II Group 5: Mask Detection and Social Distance Evaluation (slides) Group 12: Create Video Clips using Frame Interpolation (slides) Group 13: Itemization of Receipts Using Computer Vision Techniques (slides) Group 14: Real time Alertness assessment using CNN and Viola-Jones Algorithm (slides) Group 15: Parking Spot Detection (slides, demo) Group 16: YOGA Master (slides, demo1, demo2) Group 17: ZEN: A Cross architecture Generalizable Dataset Distillation Approach (slides) Group 18: naviVision (slides, demo) Group 19: Learning based 3D Representation and Rendering (slides, demo) |